Climate scenario data#
In this notebook we will…
… aggregate and download climate scenario data from the Coupled Model Intercomparison Project Phase 6 (CMIP6) for our catchment,
… preprocess the data,
… compare the CMIP6 models with our reanalysis data and adjust them for bias,
… and visualize the data before and after bias adjustment.
The NEX-GDDP-CMIP6 dataset we are going to use has been downscaled to 27830 m resolution by the NASA Climate Analytics Group and is available in two Shared Socio-Economic Pathways (SSP2 and SSP5). It is available via Google Earth Engine which makes it subsettable on the server side and the download relatively light-weight.
We start by importing and initializing the Google Earth Engine packages again.
Show code cell source
import ee
import geemap
import numpy as np
try:
ee.Initialize()
except Exception as e:
ee.Authenticate()
ee.Initialize()
The next cell reads the output directory location and the catchment outline as target polygon.
Show code cell source
import configparser
import ast
import geopandas as gpd
# read local config.ini file
config = configparser.ConfigParser()
config.read('config.ini')
# get paths from config.ini
dir_output = config['FILE_SETTINGS']['DIR_OUTPUT']
dir_figures = config['FILE_SETTINGS']['DIR_FIGURES']
output_gpkg = dir_output + config['FILE_SETTINGS']['GPKG_NAME']
# get style for matplotlib plots
plt_style = ast.literal_eval(config['CONFIG']['PLOT_STYLE'])
# set the file format for storage
compact_files = config.getboolean('CONFIG','COMPACT_FILES')
# load catchment outline as target polygon
catchment_new = gpd.read_file(output_gpkg, layer='catchment_new')
catchment = geemap.geopandas_to_ee(catchment_new)
# name target subdirectory to be created
cmip_dir = dir_output + 'cmip6/'
Select, aggregate, and download downscaled CMIP6 data#
We are going to create a class that does it all in one go. The buildFeauture()
function requests daily catchment-wide averages of all available CMIP6 models for individual years. All years requested are stored in an ee.ImageCollection
by getResult()
. To provide the best basis for bias adjustment a large overlap of reanalysis and scenario data is recommended. By default, the CMIPDownloader
class requests everything between the earliest available date from ERA5-Land (1979) and the latest available date from CMIP6 (2100). The download()
function then starts a given number of parallel requests, each downloading a single year and storing them in CSV files.
Show code cell source
import concurrent.futures
import os
import requests
from retry import retry
from tqdm import tqdm
class CMIPDownloader:
"""Class to download spatially averaged CMIP6 data for a given period, variable, and spatial subset."""
def __init__(self, var, starty, endy, shape, processes=10, dir='./'):
self.var = var
self.starty = starty
self.endy = endy
self.shape = shape
self.processes = processes
self.directory = dir
# create the download directory if it doesn't exist
if not os.path.exists(self.directory):
os.makedirs(self.directory)
def download(self):
"""Runs a subset routine for CMIP6 data on GEE servers to create ee.FeatureCollections for all years in
the requested period. Downloads individual years in parallel processes to increase the download time."""
print('Initiating download request for NEX-GDDP-CMIP6 data from ' +
str(self.starty) + ' to ' + str(self.endy) + '.')
def getRequests(starty, endy):
"""Generates a list of years to be downloaded. [Client side]"""
return [i for i in range(starty, endy+1)]
@retry(tries=10, delay=1, backoff=2)
def getResult(index, year):
"""Handle the HTTP requests to download one year of CMIP6 data. [Server side]"""
start = str(year) + '-01-01'
end = str(year + 1) + '-01-01'
startDate = ee.Date(start)
endDate = ee.Date(end)
n = endDate.difference(startDate, 'day').subtract(1)
def getImageCollection(var):
"""Create and image collection of CMIP6 data for the requested variable, period, and region.
[Server side]"""
collection = ee.ImageCollection('NASA/GDDP-CMIP6') \
.select(var) \
.filterDate(startDate, endDate) \
.filterBounds(self.shape)
return collection
def renameBandName(b):
"""Edit variable names for better readability. [Server side]"""
split = ee.String(b).split('_')
return ee.String(split.splice(split.length().subtract(2), 1).join("_"))
def buildFeature(i):
"""Create an area weighted average of the defined region for every day in the given year.
[Server side]"""
t1 = startDate.advance(i, 'day')
t2 = t1.advance(1, 'day')
# feature = ee.Feature(point)
dailyColl = collection.filterDate(t1, t2)
dailyImg = dailyColl.toBands()
# renaming and handling names
bands = dailyImg.bandNames()
renamed = bands.map(renameBandName)
# Daily extraction and adding time information
dict = dailyImg.rename(renamed).reduceRegion(
reducer=ee.Reducer.mean(),
geometry=self.shape,
).combine(
ee.Dictionary({'system:time_start': t1.millis(), 'isodate': t1.format('YYYY-MM-dd')})
)
return ee.Feature(None, dict)
# Create features for all days in the respective year. [Server side]
collection = getImageCollection(self.var)
year_feature = ee.FeatureCollection(ee.List.sequence(0, n).map(buildFeature))
# Create a download URL for a CSV containing the feature collection. [Server side]
url = year_feature.getDownloadURL()
# Handle downloading the actual csv for one year. [Client side]
r = requests.get(url, stream=True)
if r.status_code != 200:
r.raise_for_status()
filename = os.path.join(self.directory, 'cmip6_' + self.var + '_' + str(year) + '.csv')
with open(filename, 'w') as f:
f.write(r.text)
return index
# Create a list of years to be downloaded. [Client side]
items = getRequests(self.starty, self.endy)
# Launch download requests in parallel processes and display a status bar. [Client side]
with tqdm(total=len(items), desc="Downloading CMIP6 data for variable '" + self.var + "'") as pbar:
results = []
with concurrent.futures.ThreadPoolExecutor(max_workers=self.processes) as executor:
for i, year in enumerate(items):
results.append(executor.submit(getResult, i, year))
for future in concurrent.futures.as_completed(results):
index = future.result()
pbar.update(1)
print("All downloads complete.")
We can now define a target location and start the download for both desired variables individually. We choose a moderate number of requests to avoid kernel “hickups”. The download time depends on the number of parallel processes, the traffic on GEE servers, and other mysterious factors. If you run this notebook in a Binder it usually doesn’t take more than 5 min for both downloads to finish.
Show code cell source
downloader_t = CMIPDownloader(var='tas', starty=1979, endy=2100, shape=catchment, processes=30, dir=cmip_dir)
downloader_t.download()
downloader_p = CMIPDownloader(var='pr', starty=1979, endy=2100, shape=catchment, processes=30, dir=cmip_dir)
downloader_p.download()
Initiating download request for NEX-GDDP-CMIP6 data from 1979 to 2100.
Downloading CMIP6 data for variable 'tas': 100%|██████████████████████████████████████████████████████████████████████████████████████████████████████████████████████████████| 122/122 [01:13<00:00, 1.65it/s]
All downloads complete.
Initiating download request for NEX-GDDP-CMIP6 data from 1979 to 2100.
Downloading CMIP6 data for variable 'pr': 100%|███████████████████████████████████████████████████████████████████████████████████████████████████████████████████████████████| 122/122 [01:01<00:00, 1.97it/s]
All downloads complete.
We have now downloaded individual files for each year and variable and stored them in cmip_dir
. To use them as model forcing data, they need to be processed.
Process the downloaded CSV files#
The following class will read all downloaded CSV files and concatenate them to a single file per scenario. It further checks for consistency and drops models not available for individual years or scenarios.
Show code cell source
import pandas as pd
class CMIPProcessor:
"""Class to read and pre-process CSV files downloaded by the CMIPDownloader class."""
def __init__(self, var, file_dir='.'):
self.file_dir = file_dir
self.var = var
self.df_hist = self.append_df(self.var, self.file_dir, hist=True)
self.df_ssp = self.append_df(self.var, self.file_dir, hist=False)
self.ssp2_common, self.ssp5_common, self.hist_common,\
self.common_models, self.dropped_models = self.process_dataframes()
self.ssp2, self.ssp5 = self.get_results()
def read_cmip(self, filename):
"""Reads CMIP6 CSV files and drops redundant columns."""
df = pd.read_csv(filename, index_col='isodate', parse_dates=['isodate'])
df = df.drop(['system:index', '.geo', 'system:time_start'], axis=1)
return df
def append_df(self, var, file_dir='.', hist=True):
"""Reads CMIP6 CSV files of individual years and concatenates them into dataframes for the full downloaded
period. Historical and scenario datasets are treated separately. Converts precipitation unit to mm."""
df_list = []
if hist:
starty = 1979
endy = 2014
else:
starty = 2015
endy = 2100
for i in range(starty, endy + 1):
filename = file_dir + 'cmip6_' + var + '_' + str(i) + '.csv'
df_list.append(self.read_cmip(filename))
if hist:
hist_df = pd.concat(df_list)
if var == 'pr':
hist_df = hist_df * 86400 # from kg/(m^2*s) to mm/day
return hist_df
else:
ssp_df = pd.concat(df_list)
if var == 'pr':
ssp_df = ssp_df * 86400 # from kg/(m^2*s) to mm/day
return ssp_df
def process_dataframes(self):
"""Separates the two scenarios and drops models not available for both scenarios and the historical period."""
ssp2 = self.df_ssp.loc[:, self.df_ssp.columns.str.startswith('ssp245')]
ssp5 = self.df_ssp.loc[:, self.df_ssp.columns.str.startswith('ssp585')]
hist = self.df_hist.loc[:, self.df_hist.columns.str.startswith('historical')]
ssp2.columns = ssp2.columns.str.lstrip('ssp245_').str.rstrip('_' + self.var)
ssp5.columns = ssp5.columns.str.lstrip('ssp585_').str.rstrip('_' + self.var)
hist.columns = hist.columns.str.lstrip('historical_').str.rstrip('_' + self.var)
# Get all the models the three datasets have in common
common_models = set(ssp2.columns).intersection(ssp5.columns).intersection(hist.columns)
# Get the model names that contain NaN values
nan_models_list = [df.columns[df.isna().any()].tolist() for df in [ssp2, ssp5, hist]]
# flatten the list
nan_models = [col for sublist in nan_models_list for col in sublist]
# remove duplicates
nan_models = list(set(nan_models))
# Remove models with NaN values from the list of common models
common_models = [x for x in common_models if x not in nan_models]
ssp2_common = ssp2.loc[:, common_models]
ssp5_common = ssp5.loc[:, common_models]
hist_common = hist.loc[:, common_models]
dropped_models = list(set([mod for mod in ssp2.columns if mod not in common_models] +
[mod for mod in ssp5.columns if mod not in common_models] +
[mod for mod in hist.columns if mod not in common_models]))
return ssp2_common, ssp5_common, hist_common, common_models, dropped_models
def get_results(self):
"""Concatenates historical and scenario data to combined dataframes of the full downloaded period.
Arranges the models in alphabetical order."""
ssp2_full = pd.concat([self.hist_common, self.ssp2_common])
ssp2_full.index.names = ['TIMESTAMP']
ssp5_full = pd.concat([self.hist_common, self.ssp5_common])
ssp5_full.index.names = ['TIMESTAMP']
ssp2_full = ssp2_full.reindex(sorted(ssp2_full.columns), axis=1)
ssp5_full = ssp5_full.reindex(sorted(ssp5_full.columns), axis=1)
return ssp2_full, ssp5_full
The CMIPProcessor
processes variables individually and returns a single dataframe for each of both scenarios from 1979 to 2100.
Show code cell source
cmip_dir = dir_output + 'cmip6/'
processor_t = CMIPProcessor(file_dir=cmip_dir, var='tas')
ssp2_tas_raw, ssp5_tas_raw = processor_t.get_results()
processor_p = CMIPProcessor(file_dir=cmip_dir, var='pr')
ssp2_pr_raw, ssp5_pr_raw = processor_p.get_results()
Let’s have a look. We can see that our scenario dataset now contains 33 CMIP6 models in alphabetical order.
Show code cell source
print(ssp2_tas_raw.info())
<class 'pandas.core.frame.DataFrame'>
DatetimeIndex: 44560 entries, 1979-01-01 to 2100-12-31
Data columns (total 33 columns):
# Column Non-Null Count Dtype
--- ------ -------------- -----
0 ACCESS-CM2 44560 non-null float64
1 ACCESS-ESM1-5 44560 non-null float64
2 BCC-CSM2-MR 44560 non-null float64
3 CESM2 44560 non-null float64
4 CESM2-WACCM 44560 non-null float64
5 CMCC-CM2-SR5 44560 non-null float64
6 CMCC-ESM2 44560 non-null float64
7 CNRM-CM6-1 44560 non-null float64
8 CNRM-ESM2-1 44560 non-null float64
9 CanESM5 44560 non-null float64
10 EC-Earth3 44560 non-null float64
11 EC-Earth3-Veg-LR 44560 non-null float64
12 FGOALS-g3 44560 non-null float64
13 GFDL-CM4_gr1 44560 non-null float64
14 GFDL-CM4_gr2 44560 non-null float64
15 GFDL-ESM4 44560 non-null float64
16 GISS-E2-1-G 44560 non-null float64
17 HadGEM3-GC31-LL 44560 non-null float64
18 INM-CM4-8 44560 non-null float64
19 INM-CM5-0 44560 non-null float64
20 IPSL-CM6A-LR 44560 non-null float64
21 KACE-1-0-G 44560 non-null float64
22 KIOST-ESM 44560 non-null float64
23 MIROC-ES2L 44560 non-null float64
24 MIROC6 44560 non-null float64
25 MPI-ESM1-2-HR 44560 non-null float64
26 MPI-ESM1-2-LR 44560 non-null float64
27 MRI-ESM2-0 44560 non-null float64
28 NESM3 44560 non-null float64
29 NorESM2-LM 44560 non-null float64
30 NorESM2-MM 44560 non-null float64
31 TaiESM1 44560 non-null float64
32 UKESM1-0-LL 44560 non-null float64
dtypes: float64(33)
memory usage: 11.6 MB
None
If we want to check which models failed the consistency check of the CMIPProcessor
we can use its dropped_models
attribute.
Show code cell source
print(processor_t.dropped_models)
['HadGEM3-GC31-MM', 'IITM-ESM']
Bias adjustment using reananlysis data#
Due to the coarse resolution of global climate models (GCMs) and the extensive correction of reanalysis data there is substantial bias between the two datasets. To force a glacio-hydrological model calibrated on reanalysis data with climate scenarios this bias needs to be adressed. We will use a method developed by Switanek et.al. (2017) called Scaled Distribution Mapping (SDM) to correct for bias while preserving trends and the likelihood of meteorological events in the raw GCM data. The method has been implemented in the bias_correction
Python library by Pankaj Kumar.
We will first create a function to read our reanalysis CSV. The adjust_bias()
function will then loop over all models and adjust them to the reanalysis data in the overlap period (1979 to 2022).
Show code cell source
from bias_correction import BiasCorrection
def read_era5l(file):
"""Reads ERA5-Land data, drops redundant columns, and adds DatetimeIndex.
Resamples the dataframe to reduce the DatetimeIndex to daily resolution."""
return pd.read_csv(file, **{
'usecols': ['temp', 'prec', 'dt'],
'index_col': 'dt',
'parse_dates': ['dt']}).resample('D').agg({'temp': 'mean', 'prec': 'sum'})
def adjust_bias(predictand, predictor, method='normal_mapping'):
"""Applies scaled distribution mapping to all passed climate projections (predictand)
based on a predictor timeseries."""
predictor = read_era5l(predictor)
if predictand.mean().mean() > 100:
var = 'temp'
else:
var = 'prec'
training_period = slice('1979-01-01', '2022-12-31')
prediction_period = slice('1979-01-01', '2100-12-31')
corr = pd.DataFrame()
for m in predictand.columns:
x_train = predictand[m][training_period].squeeze()
y_train = predictor[training_period][var].squeeze()
x_predict = predictand[m][prediction_period].squeeze()
bc_corr = BiasCorrection(y_train, x_train, x_predict)
corr[m] = pd.DataFrame(bc_corr.correct(method=method))
return corr
The function is applied to every variable and scenario separately. The bias_adjustment
library offers a normal and a gamma distribution as basis for the SDM. As the distribution of the ERA5-Land precipitation data is actually closer to normal distribution capped at 0 mm, we use the normal_mapping
method for both variables.
Show code cell source
era5_file = dir_output + 'ERA5L.csv'
ssp2_tas = adjust_bias(predictand=ssp2_tas_raw, predictor=era5_file)
ssp5_tas = adjust_bias(predictand=ssp5_tas_raw, predictor=era5_file)
ssp2_pr = adjust_bias(predictand=ssp2_pr_raw, predictor=era5_file)
ssp5_pr = adjust_bias(predictand=ssp5_pr_raw, predictor=era5_file)
The result is a comprehensive dataset of 33 models over 122 years in two versions (pre- and post-adjustment) for every variable. To see what’s in the data and what happened during bias adjustment we need an overview.
Visualization#
First, we store our raw and adjusted data in dictionaries.
Show code cell source
ssp_tas_dict = {'SSP2_raw': ssp2_tas_raw, 'SSP2_adjusted': ssp2_tas, 'SSP5_raw': ssp5_tas_raw, 'SSP5_adjusted': ssp5_tas}
ssp_pr_dict = {'SSP2_raw': ssp2_pr_raw, 'SSP2_adjusted': ssp2_pr, 'SSP5_raw': ssp5_pr_raw, 'SSP5_adjusted': ssp5_pr}
The first plot will contain simple timeseries. The first function cmip_plot()
resamples the data so a given frequency and creates a single plot. cmip_plot_combined()
arranges multiple plots for both scenarios before and after bias adjustment.
Time series#
Show code cell source
import matplotlib.pyplot as plt
import seaborn as sns
import scienceplots
# set style from config
plt.style.use(plt_style)
def cmip_plot(ax, df, target, title=None, precip=False, intv_sum='ME', intv_mean='10YE',
target_label='Target', show_target_label=False):
"""Resamples and plots climate model and target data."""
if intv_mean == '10YE' or intv_mean == '5YE' or intv_mean == '20YE':
closure = 'left'
else:
closure = 'right'
if not precip:
ax.plot(df.resample(intv_mean, closed=closure, label='left').mean().iloc[:, :], linewidth=1.2)
era_plot, = ax.plot(target['temp'].resample(intv_mean).mean(), linewidth=1.5, c='red', label=target_label,
linestyle='dashed')
else:
ax.plot(df.resample(intv_sum).sum().resample(intv_mean, closed=closure, label='left').mean().iloc[:, :],
linewidth=1.2)
era_plot, = ax.plot(target['prec'].resample(intv_sum).sum().resample(intv_mean).mean(), linewidth=1.5,
c='red', label=target_label, linestyle='dashed')
if show_target_label:
ax.legend(handles=[era_plot], loc='upper left')
ax.set_title(title)
ax.grid(True)
def cmip_plot_combined(data, target, title=None, precip=False, intv_sum='ME', intv_mean='10YE',
target_label='Target', show=False, saveas=None):
"""Combines multiple subplots of climate data in different scenarios before and after bias adjustment.
Shows target data for comparison"""
figure, axis = plt.subplots(2, 2, figsize=(12, 12), sharex="col", sharey="all")
t_kwargs = {'target': target, 'intv_mean': intv_mean, 'target_label': target_label}
p_kwargs = {'target': target, 'intv_mean': intv_mean, 'target_label': target_label,
'intv_sum': intv_sum, 'precip': True}
if not precip:
cmip_plot(axis[0, 0], data['SSP2_raw'], show_target_label=True, title='SSP2 raw', **t_kwargs)
cmip_plot(axis[0, 1], data['SSP2_adjusted'], title='SSP2 adjusted', **t_kwargs)
cmip_plot(axis[1, 0], data['SSP5_raw'], title='SSP5 raw', **t_kwargs)
cmip_plot(axis[1, 1], data['SSP5_adjusted'], title='SSP5 adjusted', **t_kwargs)
figure.legend(data['SSP5_adjusted'].columns, loc='lower right', ncol=6, mode="expand")
figure.tight_layout()
figure.subplots_adjust(bottom=0.15, top=0.92)
figure.suptitle(title, fontweight='bold')
if saveas is not None:
plt.savefig(dir_figures + saveas)
if show:
plt.show()
else:
cmip_plot(axis[0, 0], data['SSP2_raw'], show_target_label=True, title='SSP2 raw', **p_kwargs)
cmip_plot(axis[0, 1], data['SSP2_adjusted'], title='SSP2 adjusted', **p_kwargs)
cmip_plot(axis[1, 0], data['SSP5_raw'], title='SSP5 raw', **p_kwargs)
cmip_plot(axis[1, 1], data['SSP5_adjusted'], title='SSP5 adjusted', **p_kwargs)
figure.legend(data['SSP5_adjusted'].columns, loc='lower right', ncol=6, mode="expand")
figure.tight_layout()
figure.subplots_adjust(bottom=0.15, top=0.92)
figure.suptitle(title, fontweight='bold')
if saveas is not None:
plt.savefig(dir_figures + saveas)
if show:
plt.show()
By default temperature data is resampled to 10y means (intv_mean='10YE'
), precipitation data is shown in 10y (intv_mean='10YE'
) means of monthly sums (intv_sum='ME'
). You can adapt this as you please by specifying the respective arguments.
Show code cell source
era5 = read_era5l(era5_file)
cmip_plot_combined(data=ssp_tas_dict, target=era5, title='10y Mean of Air Temperature', target_label='ERA5-Land', show=True, saveas='NB3_CMIP6_Temp.png')
cmip_plot_combined(data=ssp_pr_dict, target=era5, title='10y Mean of Monthly Precipitation', precip=True, target_label='ERA5-Land', show=True, intv_mean='10YE', saveas='NB3_CMIP6_Prec.png')
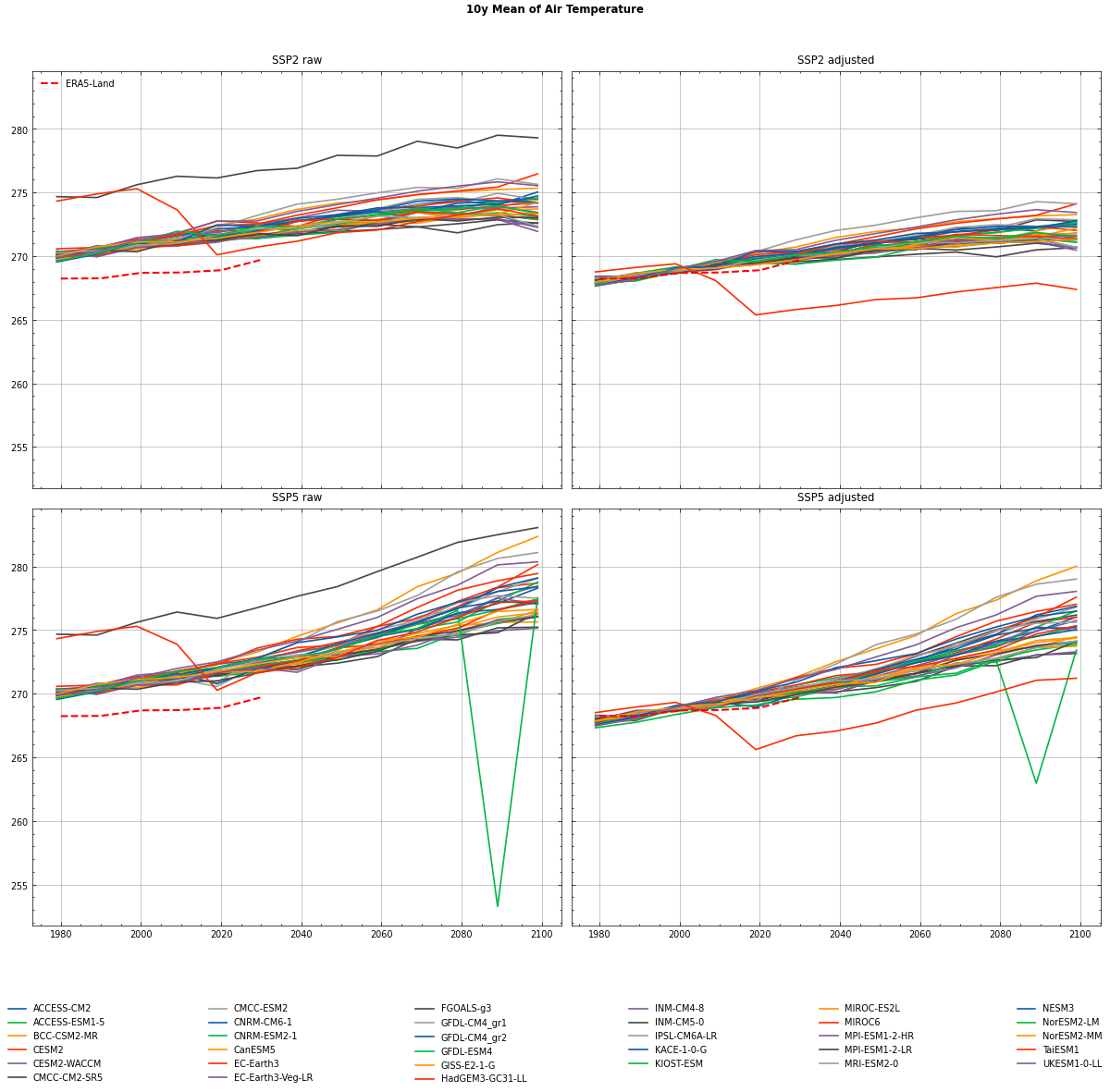
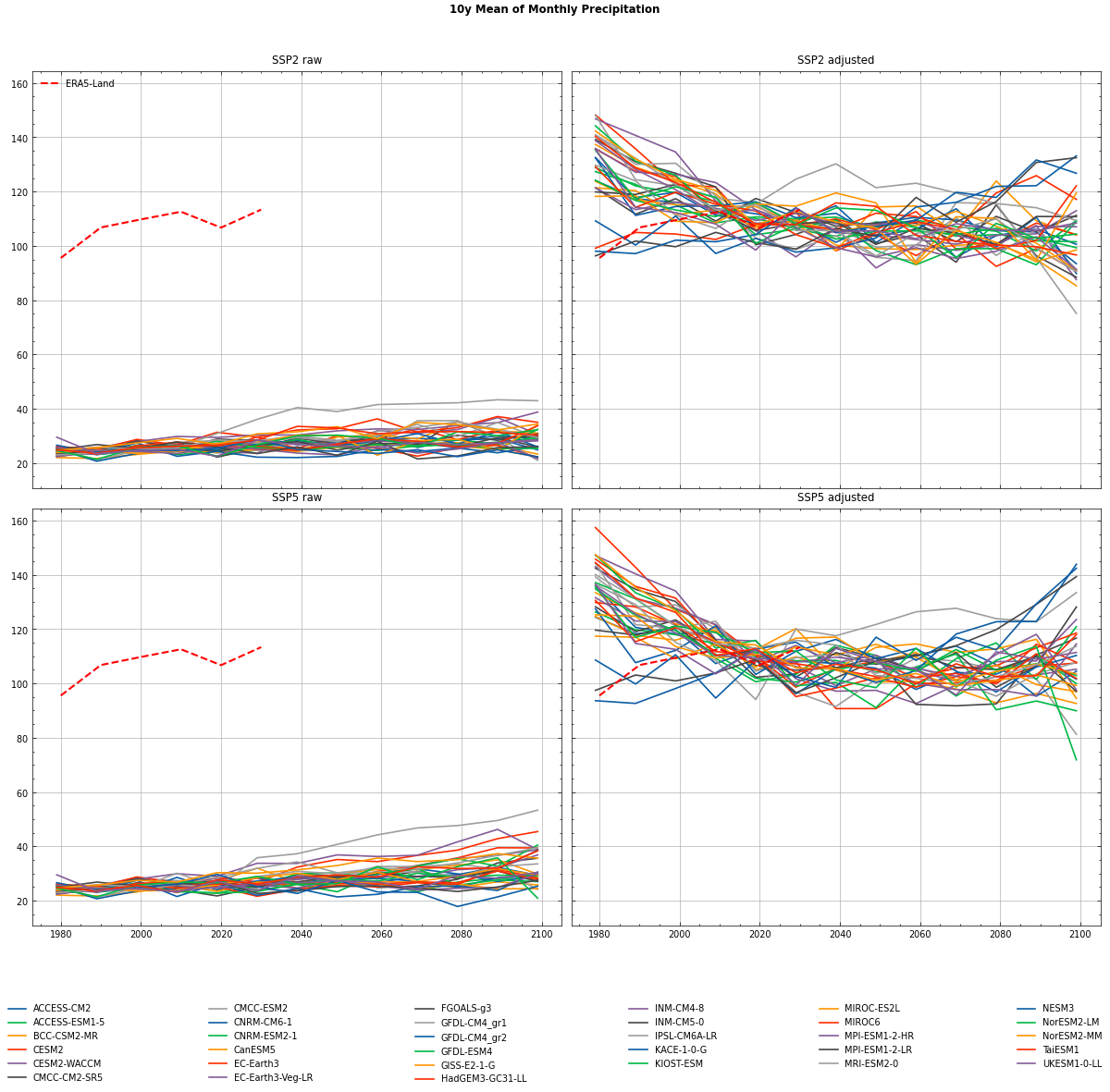
Apparently, some models have striking curves indicating unrealistic outliers or sudden jumps in the data. To clearly identify faulty time series, one option is to choose a qualitative approach by identifying the models using an interactive plotly
plot. Here we can zoom and select/deselect curves as we like, to identify model names.
Show code cell source
import plotly.express as px
fig = px.line(ssp5_tas_raw.resample('10YE', closed='left', label='left').mean())
fig.show()
Violin plots#
To look at it from a different perpective we can also have a look at the individual distributions of all models. A nice way to cover several aspects at once is to use seaborne
violinplots.
First we have to rearrange our input dictionaries a little bit.
Show code cell source
def dict_filter(dictionary, filter_string):
"""Returns a dict with all elements of the input dict that contain a filter string in their keys."""
return {key.split('_')[0]: value for key, value in dictionary.items() if filter_string in key}
tas_raw = dict_filter(ssp_tas_dict, 'raw')
tas_adjusted = dict_filter(ssp_tas_dict, 'adjusted')
pr_raw = dict_filter(ssp_pr_dict, 'raw')
pr_adjusted = dict_filter(ssp_pr_dict, 'adjusted')
The violinplot()
function requires input data in long
format.
Show code cell source
def df2long(df, intv_sum='ME', intv_mean='YE', precip=False):
"""Resamples dataframes and converts them into long format to be passed to seaborn.lineplot()."""
if precip:
df = df.resample(intv_sum).sum().resample(intv_mean).mean()
df = df.reset_index()
df = df.melt('TIMESTAMP', var_name='model', value_name='prec')
else:
df = df.resample(intv_mean).mean()
df = df.reset_index()
df = df.melt('TIMESTAMP', var_name='model', value_name='temp')
return df
For comparison the vplots()
function will arrange the plots in a similar grid as in the figures above.
Show code cell source
def vplots(before, after, target, target_label='Target', precip=False, show=False, saveas=None):
"""Creates violin plots of the kernel density estimation for all models before and after bias adjustment."""
period = slice('1979-01-01', '2022-12-31')
if precip:
var = 'prec'
var_label = 'Annual Precipitation'
unit = ' [mm]'
else:
var = 'temp'
var_label = 'Mean Annual Air Temperature'
unit = ' [K]'
for i in before.keys():
before[i] = before[i].loc[period].copy()
before[i][target_label] = target[var][period]
for i in after.keys():
after[i] = after[i].loc[period].copy()
after[i][target_label] = target[var][period]
fig = plt.figure(figsize=(20, 20))
outer = fig.add_gridspec(1, 2)
inner = outer[0].subgridspec(2, 1)
axis = inner.subplots(sharex='col')
all_data = pd.concat([df2long(before[i], precip=precip, intv_sum='YE') for i in before.keys()] +
[df2long(after[i], precip=precip, intv_sum='YE') for i in after.keys()])
xmin, xmax = all_data[var].min(), all_data[var].max()
if precip:
xlim = (xmin * 0.95, xmax * 1.05)
else:
xlim = (xmin - 1, xmax +1 )
for (i, k) in zip(before.keys(), range(0, 4, 1)):
df = df2long(before[i], precip=precip, intv_sum='YE')
axis[k].grid()
sns.violinplot(ax=axis[k], x=var, y='model', data=df, density_norm="count", bw_adjust=.2)
axis[k].set(xlim=xlim)
axis[k].set_ylabel(i, fontsize=18, fontweight='bold')
if k == 0:
axis[k].set_title('Before Scaled Distribution Mapping', fontweight='bold', fontsize=18)
plt.xlabel(var_label + unit)
inner = outer[1].subgridspec(2, 1)
axis = inner.subplots(sharex='col')
for (i, k) in zip(after.keys(), range(0, 4, 1)):
df = df2long(after[i], precip=precip, intv_sum='YE')
axis[k].grid()
sns.violinplot(ax=axis[k], x=var, y='model', data=df, density_norm="count", bw_adjust=.2)
axis[k].set(xlim=xlim)
axis[k].set_ylabel(i, fontsize=18, fontweight='bold')
axis[k].get_yaxis().set_visible(False)
if k == 0:
axis[k].set_title('After Scaled Distribution Mapping', fontweight='bold', fontsize=18)
plt.xlabel(var_label + unit)
starty = period.start.split('-')[0]
endy = period.stop.split('-')[0]
fig.suptitle('Kernel Density Estimation of ' + var_label + ' (' + starty + '-' + endy + ')',
fontweight='bold', fontsize=20)
fig.tight_layout()
fig.subplots_adjust(top=0.93)
if saveas is not None:
plt.savefig(dir_figures + saveas)
if show:
plt.show()
Again, we run the plotting function for every variable individually.
Show code cell source
vplots(tas_raw, tas_adjusted, era5, target_label='ERA5-Land', show=True, saveas='NB3_vplot_Temp.png')
vplots(pr_raw, pr_adjusted, era5, target_label='ERA5-Land', precip=True, show=True, saveas='NB3_vplot_Prec.png')
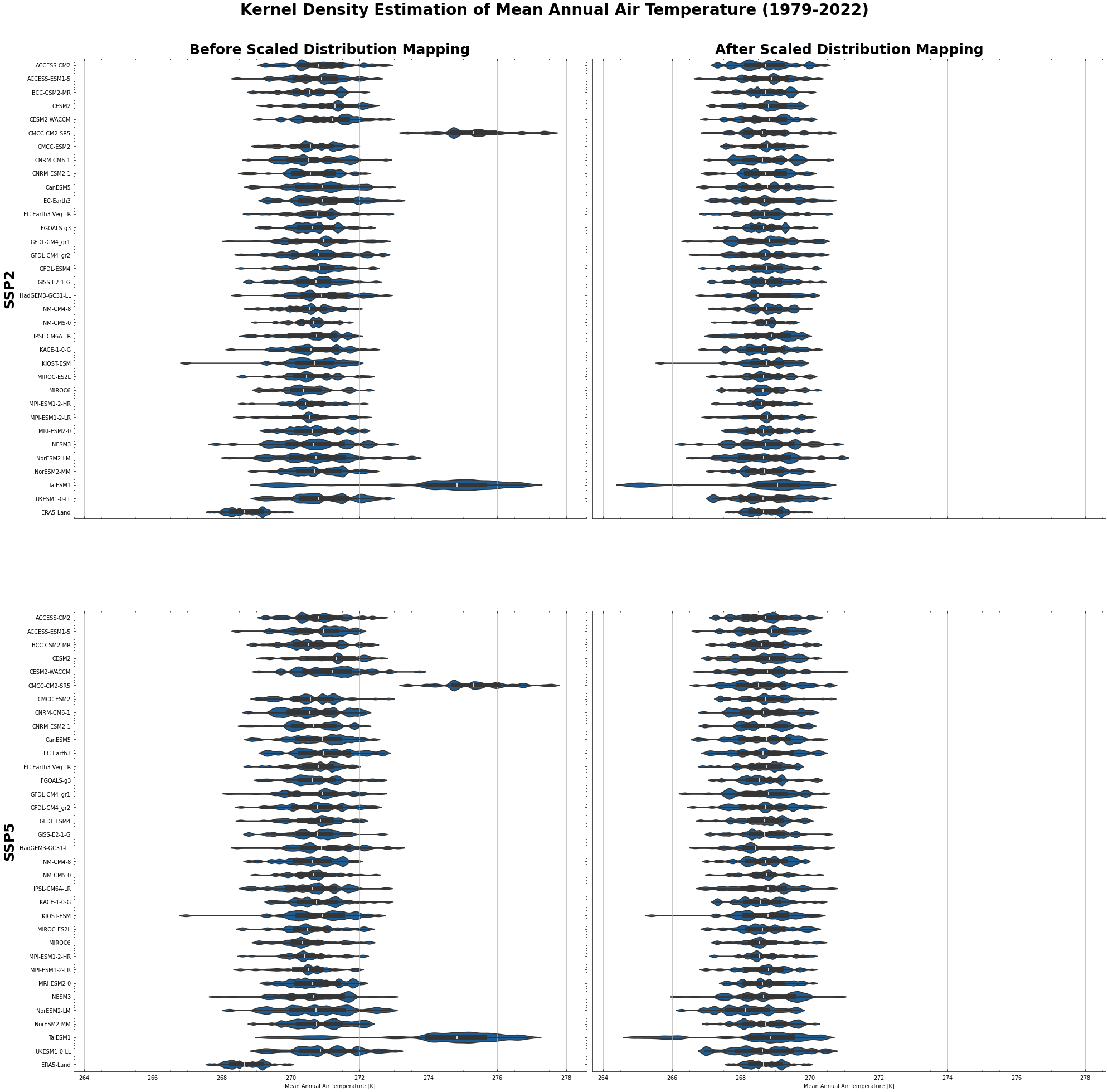
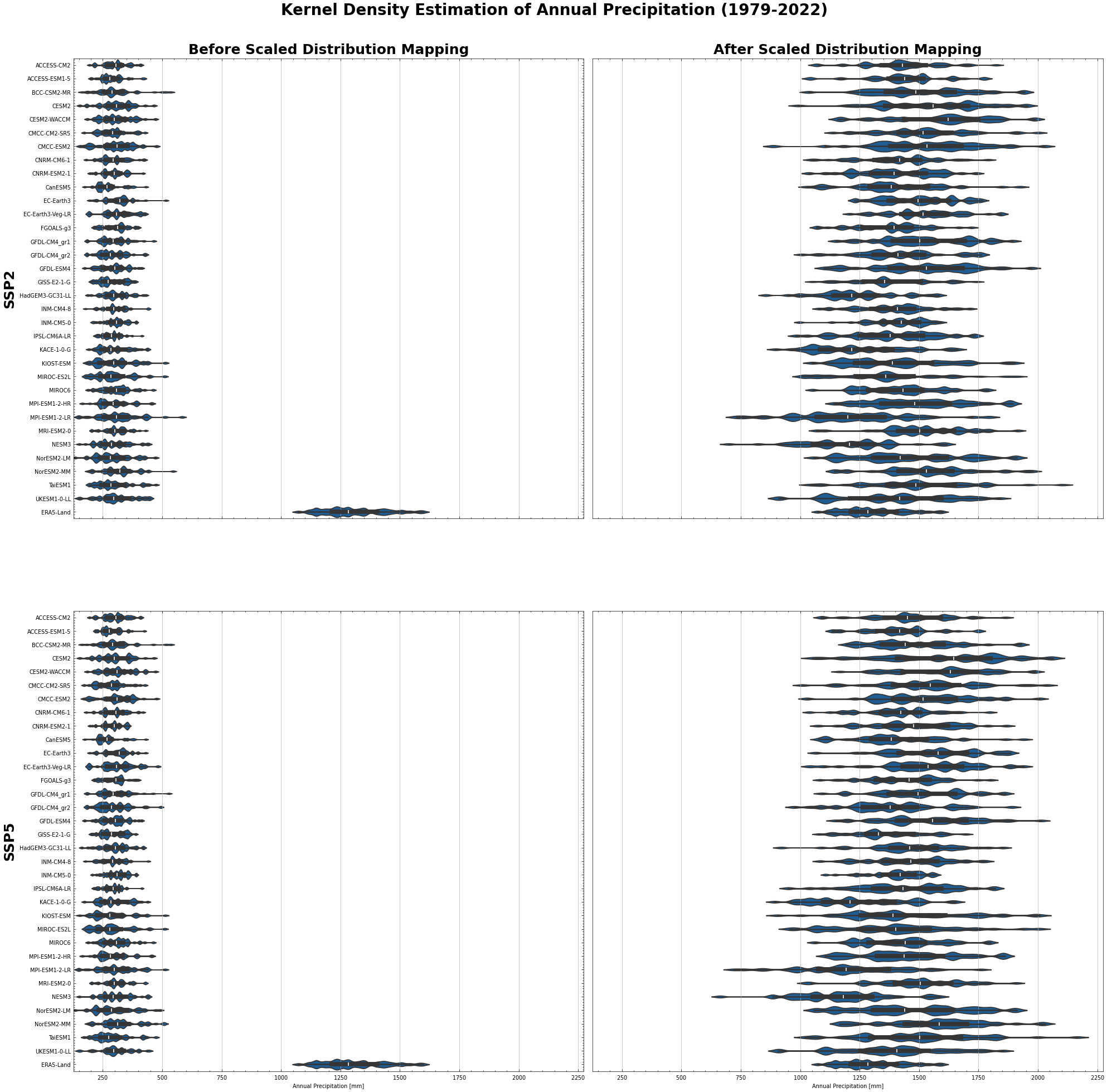
Data filters#
Alternatively, we can create some helper functions to remove respective models automatically and combine them in a handy class.
Show code cell source
class DataFilter:
def __init__(self, df, zscore_threshold=3, resampling_rate=None, prec=False, jump_threshold=5):
self.df = df
self.zscore_threshold = zscore_threshold
self.resampling_rate = resampling_rate
self.prec = prec
self.jump_threshold = jump_threshold
self.filter_all()
def check_outliers(self):
"""
A function for filtering a pandas dataframe for columns with obvious outliers
and dropping them based on a z-score threshold.
Returns
-------
models : list
A list of columns identified as having outliers.
"""
# Resample if rate specified
if self.resampling_rate is not None:
if self.prec:
self.df = self.df.resample(self.resampling_rate).sum()
else:
self.df = self.df.resample(self.resampling_rate).mean()
# Calculate z-scores for each column
z_scores = pd.DataFrame((self.df - self.df.mean()) / self.df.std())
# Identify columns with at least one outlier (|z-score| > threshold)
cols_with_outliers = z_scores.abs().apply(lambda x: any(x > self.zscore_threshold))
self.outliers = list(self.df.columns[cols_with_outliers])
# Return the list of columns with outliers
return self.outliers
def check_jumps(self):
"""
A function for checking a pandas dataframe for columns with sudden jumps or drops
and returning a list of the columns that have them.
Returns
-------
jumps : list
A list of columns identified as having sudden jumps or drops.
"""
cols = self.df.columns
jumps = []
for col in cols:
diff = self.df[col].diff()
if (abs(diff) > self.jump_threshold).any():
jumps.append(col)
self.jumps = jumps
return self.jumps
def filter_all(self):
"""
A function for filtering a dataframe for columns with obvious outliers
or sudden jumps or drops in temperature, and returning a list of the
columns that have been filtered using either or both methods.
Returns
-------
filtered_models : list
A list of columns identified as having outliers or sudden jumps/drops in temperature.
"""
self.check_outliers()
self.check_jumps()
self.filtered_models = list(set(self.outliers) | set(self.jumps))
return self.filtered_models
By default the DataFilter
class filters models containing …
… outliers deviating more than 3 standard deviations from the mean (zscore_threshold
) and/or …
… increases/decreases of more than 5 units in a single timestep (jump_threshold
).
The functions can be applied separately (check_outliers
or check_jumps
) or together (filter_all
). All three return a list
of model names.
Here, we also use the resampling_rate
parameter to resample the data to annual means ('YE'
) before running the checks.
Show code cell source
filter = DataFilter(ssp5_tas_raw, zscore_threshold=3, jump_threshold=5, resampling_rate='YE')
print('Models with temperature outliers: ' + str(filter.outliers))
print('Models with temperature jumps: ' + str(filter.jumps))
print('Models with either one or both: ' + str(filter.filtered_models))
Models with temperature outliers: ['NorESM2-LM']
Models with temperature jumps: ['NorESM2-LM', 'TaiESM1']
Models with either one or both: ['NorESM2-LM', 'TaiESM1']
The identified columns can then be removed from the CMIP6 ensemble dataset using another helper function.
Show code cell source
def drop_model(col_names, dict_or_df):
"""
Drop columns with given names from either a dictionary of dataframes
or a single dataframe.
Parameters
----------
col_names : list of str
The list of model names to drop.
dict_or_df : dict of pandas.DataFrame or pandas.DataFrame
If a dict of dataframes, all dataframes in the dict will be edited.
If a single dataframe, only that dataframe will be edited.
Returns
-------
dict_of_dfs : dict of pandas.DataFrame or pandas.DataFrame
The updated dictionary of dataframes or dataframe with dropped columns.
"""
if isinstance(dict_or_df, dict):
# loop through the dictionary and edit each dataframe
for key in dict_or_df.keys():
if all(col_name in dict_or_df[key].columns for col_name in col_names):
dict_or_df[key] = dict_or_df[key].drop(columns=col_names)
return dict_or_df
elif isinstance(dict_or_df, pd.DataFrame):
# edit the single dataframe
if all(col_name in dict_or_df.columns for col_name in col_names):
return dict_or_df.drop(columns=col_names)
else:
raise TypeError('Input must be a dictionary or a dataframe')
We run the drop_model()
function on the dictionaries of all variables and run cmip_plot_combined()
again to check the result.
Show code cell source
ssp_tas_dict = drop_model(filter.filtered_models, ssp_tas_dict)
ssp_pr_dict = drop_model(filter.filtered_models, ssp_pr_dict)
cmip_plot_combined(data=ssp_tas_dict, target=era5, title='10y Mean of Air Temperature', target_label='ERA5-Land', show=True, saveas='NB3_CMIP6_Temp_filtered.png')
cmip_plot_combined(data=ssp_pr_dict, target=era5, title='10y Mean of Monthly Precipitation', precip=True, target_label='ERA5-Land', show=True, intv_mean='10YE', saveas='NB3_CMIP6_Prec_filtered.png')
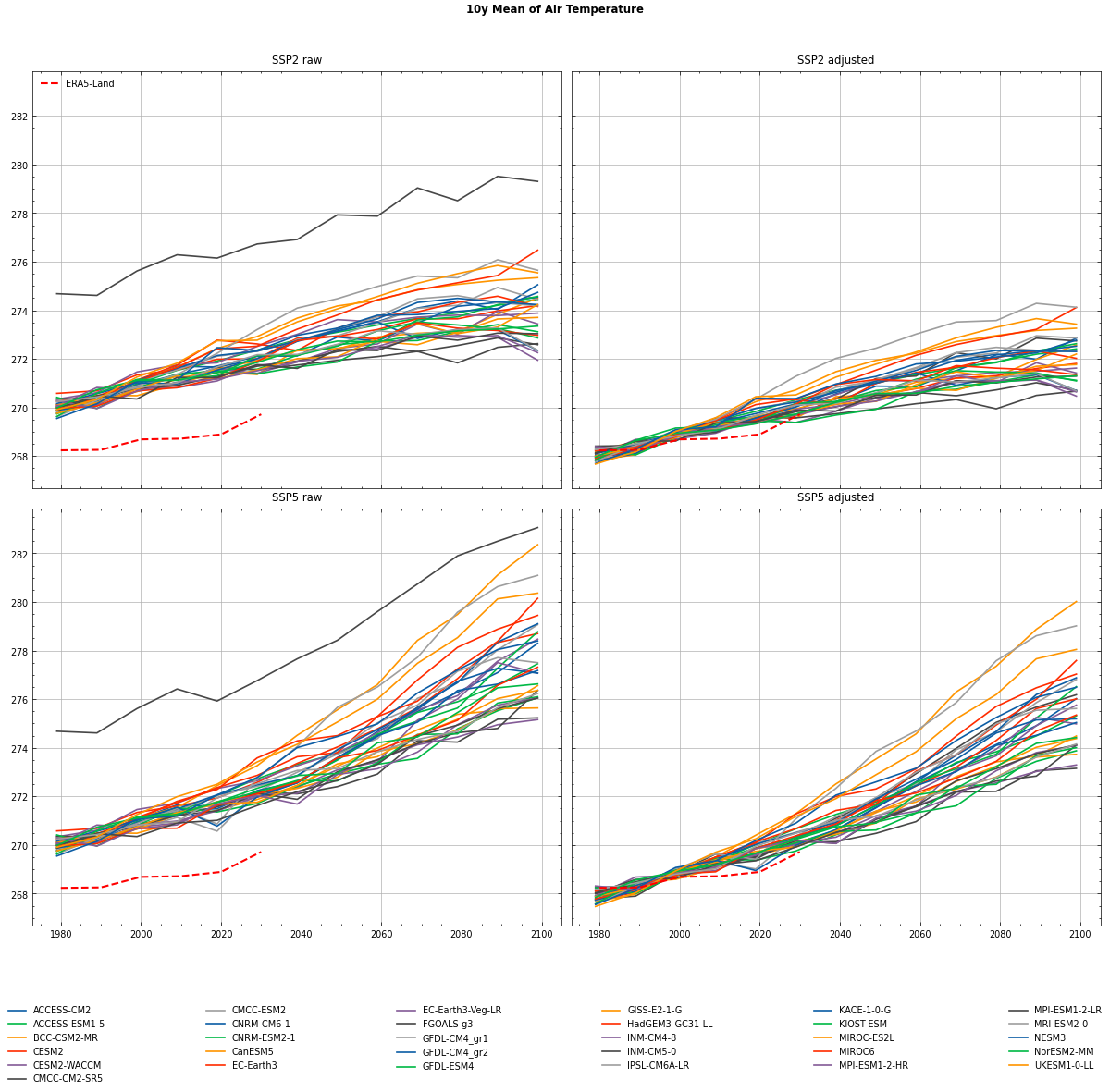
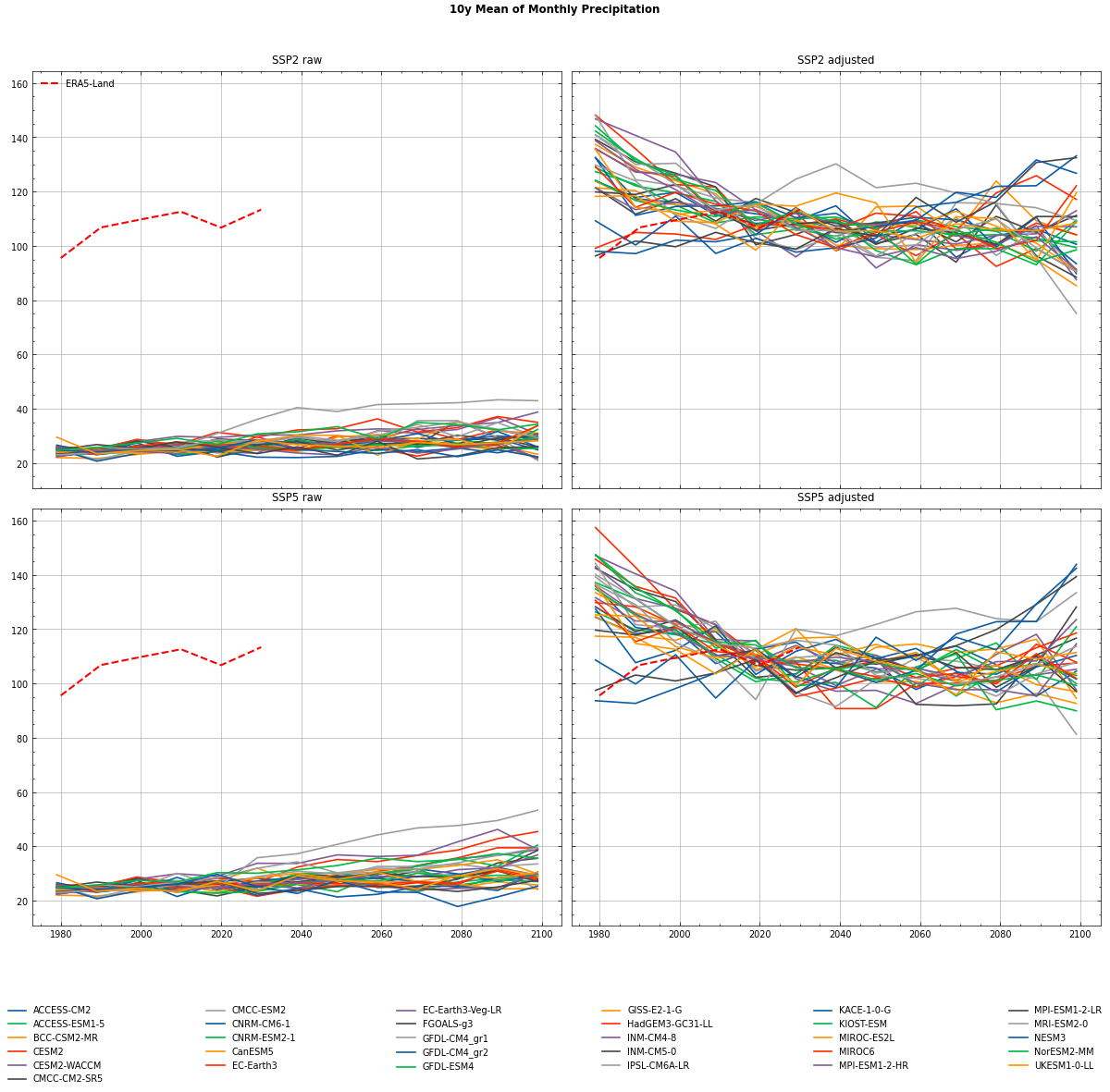
Ensemble mean plots#
As we now don’t need to focus on individual models anymore, we can reduce the number of lines by only plotting the ensemble means with a 90% confidence interval.
Show code cell source
import warnings
from matplotlib.legend import Legend
def cmip_plot_ensemble(cmip, target, precip=False, intv_sum='ME', intv_mean='YE', figsize=(10, 6), show=True, saveas=None):
"""
Plots the multi-model mean of climate scenarios including the 90% confidence interval.
Parameters
----------
cmip: dict
A dictionary with keys representing the different CMIP6 models and scenarios as pandas dataframes
containing data of temperature and/or precipitation.
target: pandas.DataFrame
Dataframe containing the historical reanalysis data.
precip: bool
If True, plot the mean precipitation. If False, plot the mean temperature. Default is False.
intv_sum: str
Interval for precipitation sums. Default is monthly ('ME').
intv_mean: str
Interval for the mean of temperature data or precipitation sums. Default is annual ('YE').
figsize: tuple
Figure size for the plot. Default is (10,6).
show: bool
If True, show the resulting plot. If False, do not show it. Default is True.
"""
warnings.filterwarnings(action='ignore')
figure, axis = plt.subplots(figsize=figsize)
# Define color palette
colors = ['darkorange', 'orange', 'darkblue', 'dodgerblue']
# create a new dictionary with the same keys but new values from the list
col_dict = {key: value for key, value in zip(cmip.keys(), colors)}
if precip:
for i in cmip.keys():
df = df2long(cmip[i], intv_sum=intv_sum, intv_mean=intv_mean, precip=True)
sns.lineplot(data=df, x='TIMESTAMP', y='prec', color=col_dict[i])
axis.set(xlabel='Year', ylabel='Mean Precipitation [mm]')
if intv_sum=='M':
figure.suptitle('Mean Monthly Precipitation', fontweight='bold')
elif intv_sum=='Y':
figure.suptitle('Mean Annual Precipitation', fontweight='bold')
target_plot = axis.plot(target.resample(intv_sum).sum().resample(intv_mean).mean(), linewidth=1.5, c='black',
label='ERA5', linestyle='dashed')
else:
for i in cmip.keys():
df = df2long(cmip[i], intv_mean=intv_mean)
sns.lineplot(data=df, x='TIMESTAMP', y='temp', color=col_dict[i])
axis.set(xlabel='Year', ylabel='Mean Air Temperature [K]')
if intv_mean=='10Y':
figure.suptitle('Mean 10y Air Temperature', fontweight='bold')
elif intv_mean == 'Y':
figure.suptitle('Mean Annual Air Temperature', fontweight='bold')
elif intv_mean == 'M':
figure.suptitle('Mean Monthly Air Temperature', fontweight='bold')
target_plot = axis.plot(target.resample(intv_mean).mean(), linewidth=1.5, c='black',
label='ERA5', linestyle='dashed')
axis.legend(['SSP2_raw', '_ci1', 'SSP2_adjusted', '_ci2', 'SSP5_raw', '_ci3', 'SSP5_adjusted', '_ci4'],
loc="upper center", bbox_to_anchor=(0.43, -0.15), ncol=4,
frameon=False) # First legend --> Workaround as seaborn lists CIs in legend
leg = Legend(axis, target_plot, ['ERA5L'], loc='upper center', bbox_to_anchor=(0.83, -0.15), ncol=1,
frameon=False) # Second legend (ERA5)
axis.add_artist(leg)
plt.grid()
figure.tight_layout(rect=[0, 0.02, 1, 1]) # Make some room at the bottom
if saveas is not None:
plt.savefig(dir_figures+saveas)
if show:
plt.show()
warnings.filterwarnings(action='always')
With less lines in the plot, we can also reduce the resample frequency and show annual means.
Show code cell source
cmip_plot_ensemble(ssp_tas_dict, era5['temp'], intv_mean='YE', saveas='NB3_CMIP6_Ensemble_Temp.png')
cmip_plot_ensemble(ssp_pr_dict, era5['prec'], precip=True, intv_sum='ME', intv_mean='YE', saveas='NB3_CMIP6_Ensemble_Prec.png')
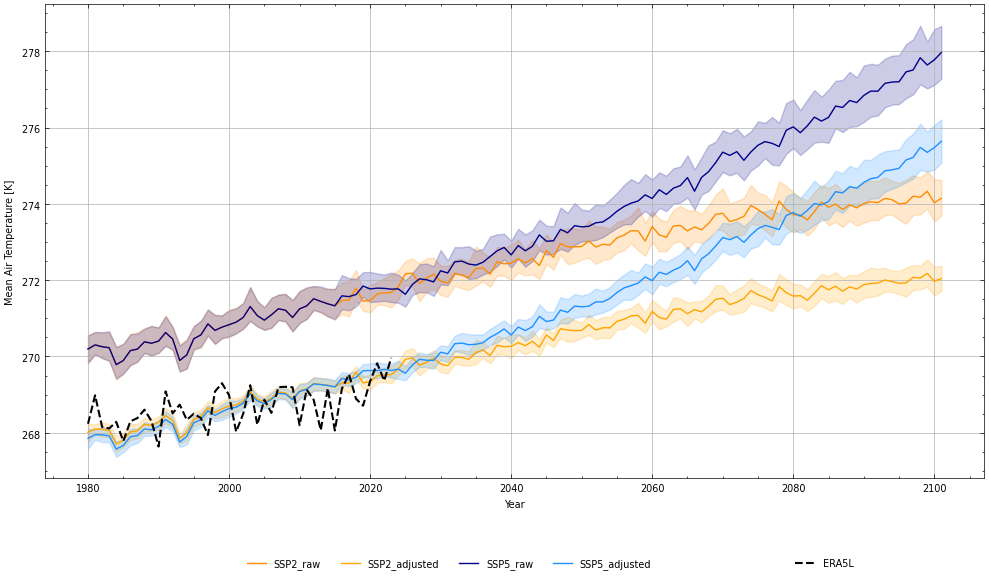
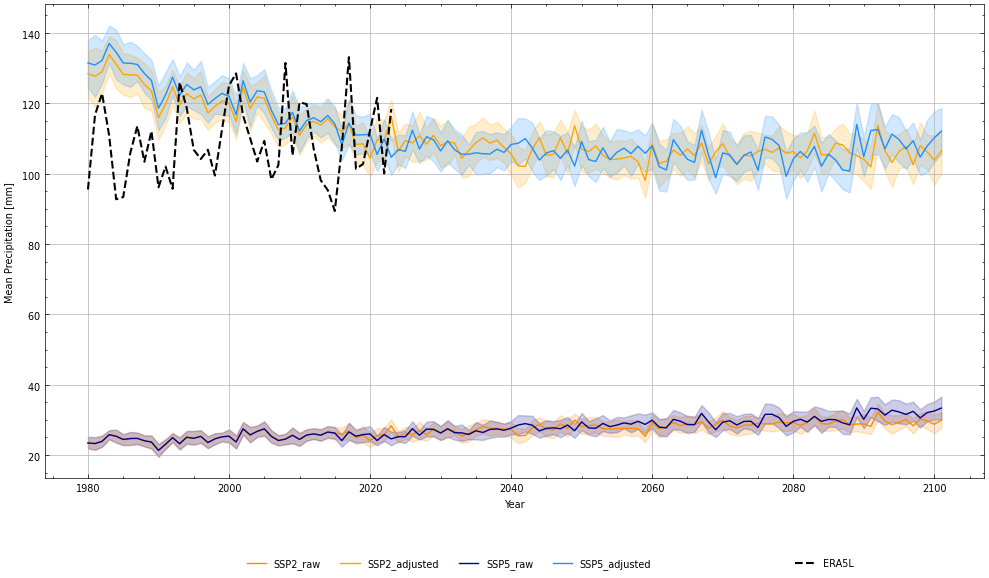
We can see that the SDM adjusts the range and mean of the target data while preserving the distribution and trend of the original data. However, the inter-model variance is slightly reduced for temperature and significantly increased for precipitation.
Last but not least, we will have a closer look at the performance of the bias adjustment. To do that, we will create probability plots for all models comparing original, target, and adjusted data with each other and a standard normal distribution. The prob_plot
function creates such a plot for an individual model and scenario. The pp_matrix
function loops the prob_plot
function over all models in a DataFrame
and arranges them in a matrix.
Show code cell source
import probscale
def prob_plot(original, target, corrected, ax, title=None, ylabel="Temperature [K]", **kwargs):
"""
Combines probability plots of climate model data before and after bias adjustment
and the target data.
Parameters
----------
original : pandas.DataFrame
The original climate model data.
target : pandas.DataFrame
The target data.
corrected : pandas.DataFrame
The climate model data after bias adjustment.
ax : matplotlib.axes.Axes
The axes on which to plot the probability plot.
title : str, optional
The title of the plot. Default is None.
ylabel : str, optional
The label for the y-axis. Default is "Temperature [K]".
**kwargs : dict, optional
Additional keyword arguments passed to the probscale.probplot() function.
Returns
-------
fig : matplotlib Figure
The generated figure.
"""
scatter_kws = dict(label="", marker=None, linestyle="-")
common_opts = dict(plottype="qq", problabel="", datalabel="", **kwargs)
scatter_kws["label"] = "original"
fig = probscale.probplot(original, ax=ax, scatter_kws=scatter_kws, **common_opts)
scatter_kws["label"] = "target"
fig = probscale.probplot(target, ax=ax, scatter_kws=scatter_kws, **common_opts)
scatter_kws["label"] = "adjusted"
fig = probscale.probplot(corrected, ax=ax, scatter_kws=scatter_kws, **common_opts)
ax.set_title(title)
ax.set_xlabel("Standard Normal Quantiles")
ax.set_ylabel(ylabel)
ax.grid(True)
score = round(target.corr(corrected), 2)
ax.text(0.05, 0.8, f"R² = {score}", transform=ax.transAxes, fontsize=15)
return fig
def pp_matrix(original, target, corrected, scenario=None, nrow=7, ncol=5, precip=False, show=False, saveas=None):
"""
Arranges the prob_plots of all CMIP6 models in a matrix and adds the R² score.
Parameters
----------
original : pandas.DataFrame
The original climate model data.
target : pandas.DataFrame
The target data.
corrected : pandas.DataFrame
The climate model data after bias adjustment.
scenario : str, optional
The climate scenario to be added to the plot title.
nrow : int, optional
The number of rows in the plot matrix. Default is 7.
ncol : int, optional
The number of columns in the plot matrix. Default is 5.
precip : bool, optional
Indicates whether the data is precipitation data. Default is False.
show : bool, optional
Indicates whether to display the plot. Default is False.
Returns
-------
None
"""
period = slice('1979-01-01', '2022-12-31')
if precip:
var = 'Precipitation'
var_label = 'Monthly ' + var
unit = ' [mm]'
original = original.resample('ME').sum()
target = target.resample('ME').sum()
corrected = corrected.resample('ME').sum()
else:
var = 'Temperature'
var_label = 'Daily Mean ' + var
unit = ' [K]'
fig = plt.figure(figsize=(16, 16))
for i, col in enumerate(original.columns):
ax = plt.subplot(nrow, ncol, i + 1)
prob_plot(original[col][period], target[period],
corrected[col][period], ax=ax, ylabel=var + unit)
ax.set_title(col, fontweight='bold')
handles, labels = ax.get_legend_handles_labels()
fig.legend(handles, ['original (CMIP6 raw)', 'target (ERA5-Land)', 'adjusted (CMIP6 after SDM)'], loc='lower right',
bbox_to_anchor=(0.96, 0.024), fontsize=20)
plt.tight_layout()
fig.subplots_adjust(hspace=0.7, wspace=0.4)
starty = period.start.split('-')[0]
endy = period.stop.split('-')[0]
if scenario is None:
fig.suptitle('Probability Plots of CMIP6 and ERA5-Land ' + var_label + ' (' + starty + '-' + endy + ')',
fontweight='bold', fontsize=20)
else:
fig.suptitle('Probability Plots of CMIP6 (' + scenario + ') and ERA5-Land ' + var_label +
' (' + starty + '-' + endy + ')', fontweight='bold', fontsize=20)
plt.subplots_adjust(top=0.93)
if saveas is not None:
plt.savefig(dir_figures+saveas)
if show:
plt.show()
First we’ll have a look at the temperature.
Show code cell source
pp_matrix(ssp2_tas_raw, era5['temp'], ssp2_tas, scenario='SSP2', show=True, saveas='NB3_CMIP6_SSP2_probability_Temp.png')
pp_matrix(ssp5_tas_raw, era5['temp'], ssp5_tas, scenario='SSP5', show=True, saveas='NB3_CMIP6_SSP5_probability_Temp.png')
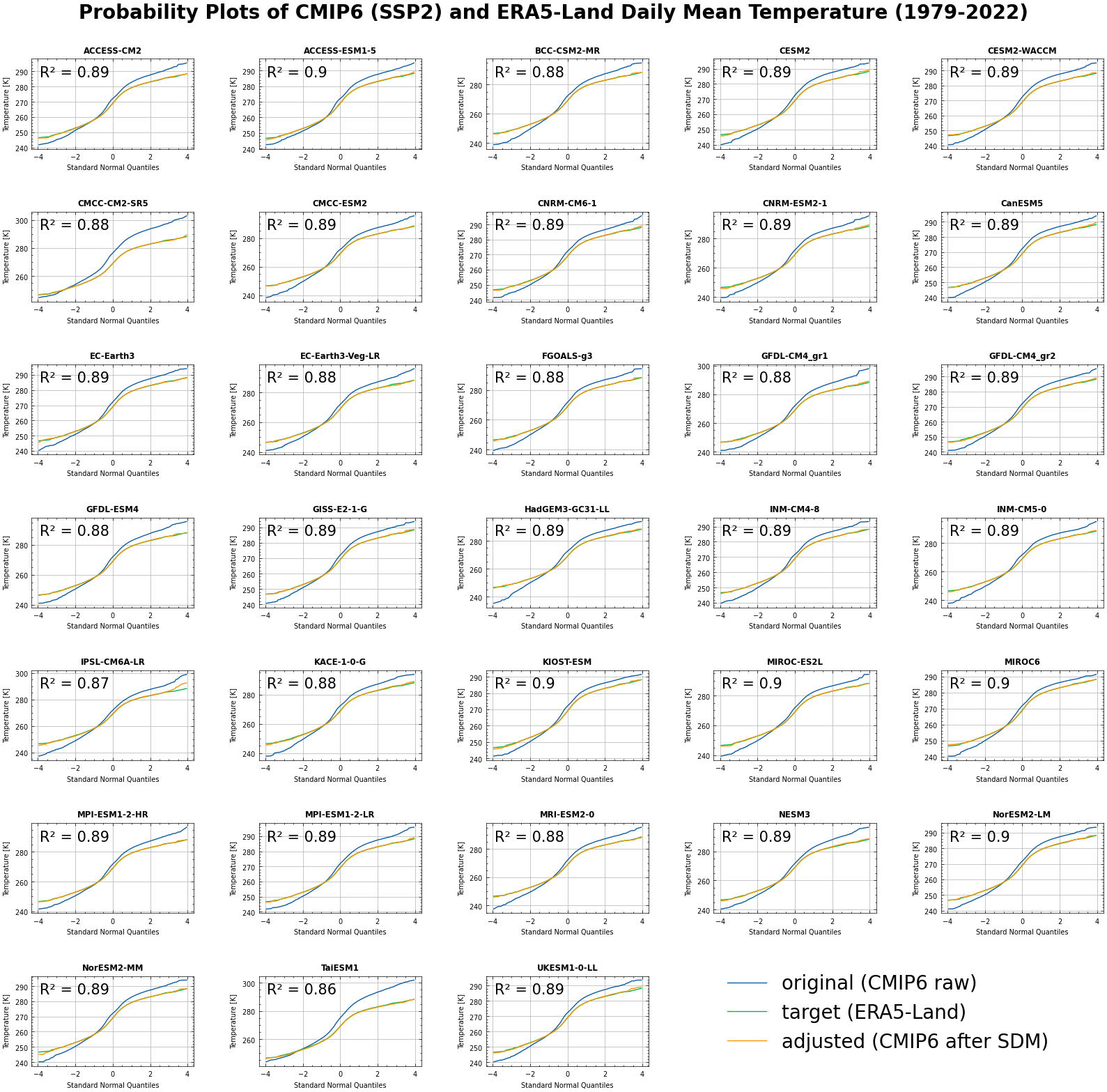
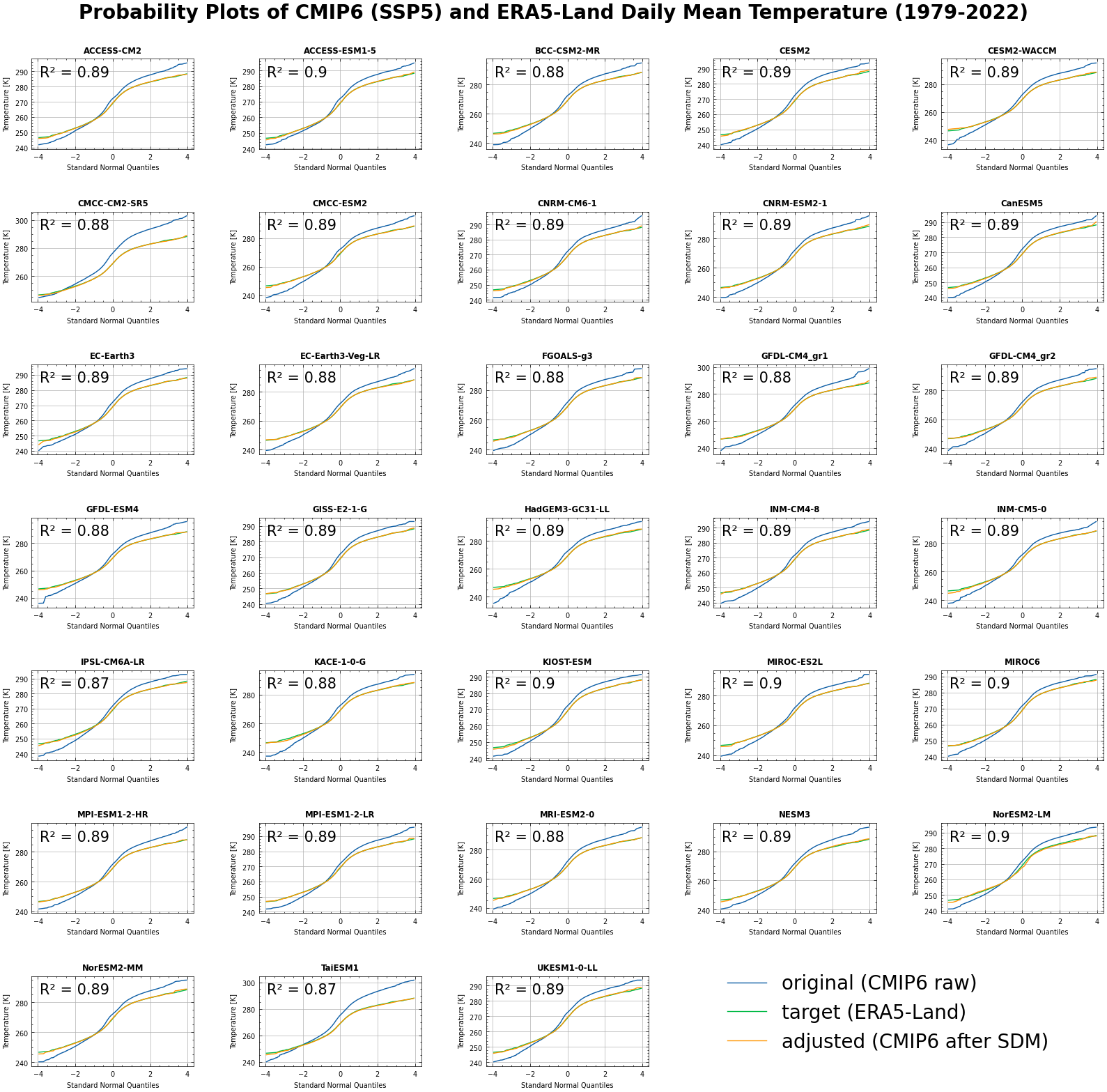
We can see that the SDM worked very well for the temperature data, with high agreement between the target and adjusted data.
Let’s look at the probability curves for precipitation. Since the precipitation data is bounded at 0, but most days have very small values >0 mm, we resample the data to monthly sums to get an idea of the overall performance.
Show code cell source
pp_matrix(ssp2_pr_raw, era5['prec'], ssp2_pr, precip=True, scenario='SSP2', show=True, saveas='NB3_CMIP6_SSP2_probability_Prec.png')
pp_matrix(ssp5_pr_raw, era5['prec'], ssp5_pr, precip=True, scenario='SSP5', show=True, saveas='NB3_CMIP6_SSP5_probability_Prec.png')
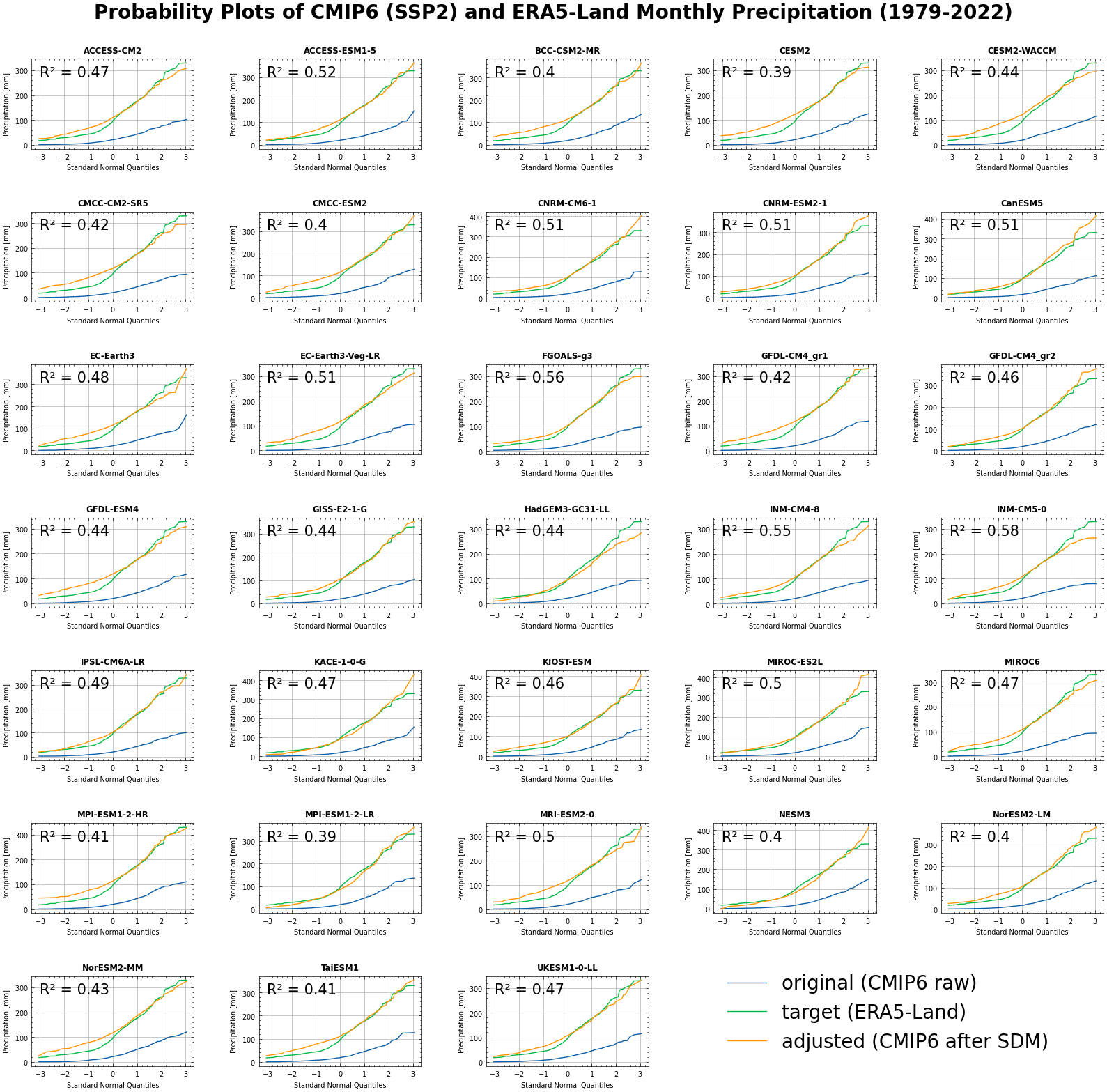
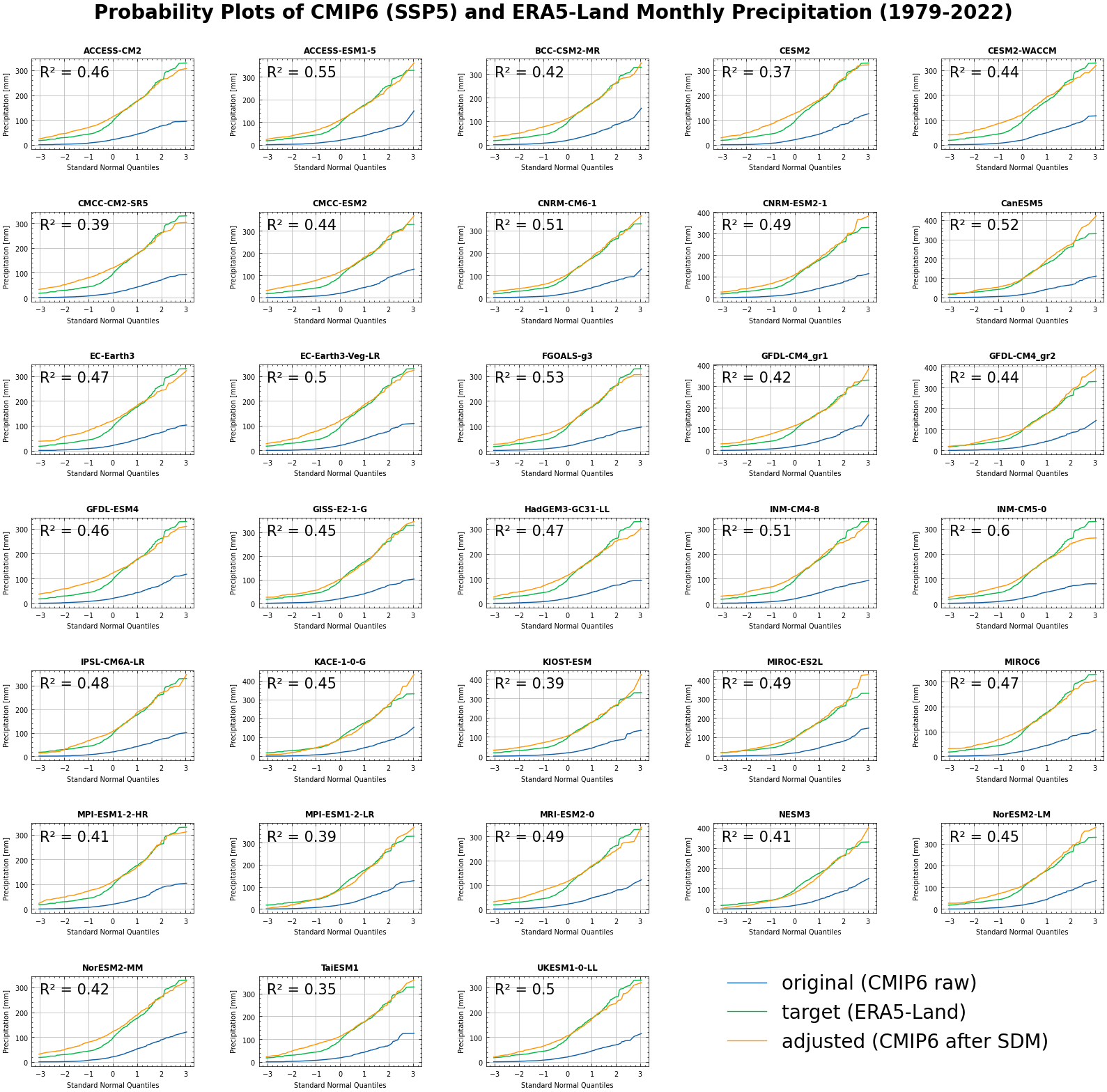
Considering the complexity and heterogeneity of precipitation data, the performance of SDM is convincing. While the fitted data of most models deviate from the target data for low and very high values, the general distribution of monthly precipitation is well met.
Write CMIP6 data to file#
After a thorough review of the climate scenario data, we can write the final selection to files for use in the next notebook. Since the whole ensemble results in relatively large files, we store the dictionaries in binary files. These are not human readable, but compact and fast to read and write.
pickle
files are fast to read and write, but take up more disk space (COMPACT_FILES = False
). You can use them on your local machine. parquet
files need less disk space but take longer to read and write (COMPACT_FILES = True
). They should be your choice in the Binder.Show code cell source
ssp_tas_dict.keys()
dict_keys(['SSP2_raw', 'SSP2_adjusted', 'SSP5_raw', 'SSP5_adjusted'])
Show code cell source
from tools.helpers import dict_to_pickle, dict_to_parquet
tas = {'SSP2': ssp_tas_dict['SSP2_adjusted'], 'SSP5': ssp_tas_dict['SSP5_adjusted']}
pr = {'SSP2': ssp_pr_dict['SSP2_adjusted'], 'SSP5': ssp_pr_dict['SSP5_adjusted']}
if compact_files:
# For storage efficiency:
dict_to_parquet(tas, cmip_dir + 'adjusted/tas_parquet')
dict_to_parquet(pr, cmip_dir + 'adjusted/pr_parquet')
else:
# For speed:
dict_to_pickle(tas, cmip_dir + 'adjusted/tas.pickle')
dict_to_pickle(pr, cmip_dir + 'adjusted/pr.pickle')
Show code cell source
import shutil
# refresh `output_download.zip` with data retrieved within this notebook
shutil.make_archive('output_download', 'zip', 'output')
print('Output folder can be download now (file output_download.zip)')
Output folder can be download now (file output_download.zip)
Show code cell source
%reset -f